PCUBE - The probability of hydrocarbons from seismic data
PCUBE - The probability of hydrocarbons from seismic data
We compute the probabilities for different lithology-fluid classes from seismic data and geological knowledge. The method combines stochastic rock physics relations between the elastic parameters and the different lithology-fluid classes, (Avseth et al., 2005), with the results from a fast Bayesian seismic simultaneous inversion from seismic data to elastic parameters (Buland and Omre, 2003). The methodological development provides a consistent framework for integrating knowledge generated by different disciplines within most oil companies. Thus it enables the maximum synergy.
Figure: The relation between different lithology-fluid classes and the elastic parameters are based on stochastic rock physics.
In the simultaneous inversion several angle stacks are inverted simultaneously
Figure: Seismic data input consist of multiple angle stacks, here near, medium and far.
From these inputs, we compute the probability of hydrocarbon presence.
Figure: The probability of hydrocarbon computed for a prospect offshore Norway.
For a prospect offshore Norway the analysis increases the probability for gas sand from 0.1 to about 0.2-0.4 in a region consistent with structural interpretation.
Figure: The match with the probability of hydrocarbons and the structural map
Due to the Bayesian formalism it is also possible to compute the expected impact of seismic data before it is collected. The method can therefore be used in feasibility studies. We compute the ability to separate lithology-fluid classes both for the elastic parameters and the inverted elastic parameters. Thus we see the loss of information due to the fact that seismic data does not resolve the elastic parameters perfectly.
Figure: Separation of lithology-fluid classes. The average predicted probability for the lithology fluid class in the columns, when the true lithology fluid class is given by the rows. The table to the left is the ability to discriminate if the elastic parameters are known; this is the best case of what we can achieve after the inversion. In the middle pane we show the prior probabilities, this is the worst case of what we can achieve after the inversion. To the right is the separation we got with the three angle stacks.
Publications
Avseth, P., T. Mukerji, and G. Mavko, 2005, Quantitative Seismic Interpretation: Cambridge University Press.
Buland, A., and H. Omre, 2003, Bayesian linearized AVO inversion: Geophysics, 68, 185-198
Buland, A., Kolbjørnsen, O. Hauge, R., Skjæveland, Ø., and Duffaut, K, (2008) Bayesian lithology and fluid prediction from seismic prestack data: Geophysics 2008 ;Volum 73.(3) s. C13-C21
Riise, O., Elgenes, J., Frey-Martinez, J.M., Kjøsnes, Ø and Buland, A., (2012) Detailed Lithology and Fluid Mapping of the Asterix Gas Discovery Using Bayesian Inversion Methodology: Proc. EAGE 2012
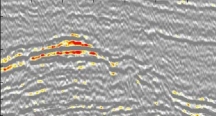