GEOPARD
GEOPARD
Geostatistical Event-based Objectmodel Predicted from Analogue Reservoir Deposits
GEOPARD is a knowledge-buildling project for industry funded by the Research Council of Norway under the PETROMAKS2 program together with industry partners Equinor Energy AS, AkerBP ASA and Winthershall Dea Norge, with special focus on improved reservoir understanding and management. Our academic partners are Professor Jo Eidsvik at Department at Mathematical Sciences at NTNU, Associate Professor Christian Haug Eide at Department of Earth Science at University of Bergen and Professor John Howell at University of Aberdeen.
The main goal of this project is to create numerical 3D models of the subsurface that integrate geological rules into the statistical modelling algorithm, and thereby
1) produce geologically meaningful model predictions,
2) allow efficient testing of different geological scenarios to improve understanding of subsurface architecture, and
3) increase the value of reservoir data and analogue field data. Improved understanding of the overall architecture in the subsurface allows for more reliable predictions of the fluid flow, and can therefore lead to optimized drainage strategies and increased recovery.
The GEOPARD project is about bringing more geological realism into the statistical framework used to generate 3D models of geology. We want to make the models easier to use and open up for direct use of data that is often overlooked. The GEOPARD project aims to formulate geological rules in a statistical framework. This mimics the geological processes by describing the geometries and distribution of the rocks, without going into the details of the physical equations. By keeping everything within a statistical framework, we can use existing algorithms for handling data conditioning and will thus be able to identify those possible reservoirs that honour both the geological concept and the observed data.
New models with more geological realism make sure that we can estimate values that will be used in the models directly from geological outcrop data already available in large databases. This will make the models easier to use, while also being aligned with both geological principles and reservoir observations.
To succeed in this interdisciplinary project, we have joined the forces of experts within the fields of geoscience, statistics, and software development, whom together with industry professionals form a resourceful team embarking on this research project to integrate geology and statistics. The project is funding one PhD in statistics at NTNU and one PostDoc in geology at University of Bergen.
Motivation:
GEOPARD is motivated by the need for more geological realism when translating from the geological conceptual model into a statistical modelling framework.
To achieve accurate reservoir predictions, the industry is in need of a facies model that can:
- Reproduce geological conceptual models consistent with reservoir observations.
- Quantify uncertainties and integrate data from a wide range of sources.
- Run as part of a reservoir simulation workflow.
Based on these needs, our primary project objective is to develop a new facies modelling algorithm conditioning to both geological rules and reservoir data. To achieve this, our secondary research objectives are to (a) define a set of geological rules, that (b) can be formulated in a Bayesian statistical framework, where (c) input parameters can be estimated from geological analogues, and (d) implement this algorithm balancing geological realism and statistical consistency.
Bayesian statistics is a proven theoretical framework for handling uncertainty and integrating data from different sources in a logically consistent and theoretically justified way. Geological knowledge gives us rules that help us describe realistic facies relationships. Geological rules can be for example the stacking pattern of shallow marine parasequences, accretion directions of fluvial channels, or density of sand packages in deep marine deposits. GEOPARD is about tying these scientific domains together to meet the industry demands.
The research questions:
- Q1: What geological rules represent depositional environments?
- Q2: How to specify geological rules in a Bayesian statistical framework?
- Q3: Which input parameters can be estimated from geological analogues?
- Q4: How to implement data conditioning algorithms in a rule-based facies model?
Q1 - What geological rules represent the depositional environments?
The main goal of this work package is to extract general rules for a range of common depositional environments based on synthesizing database information, existing literature and targeted field work. Success in this work package will be to provide a set of rules that result in the generation of geologically realistic 3D facies models.
Primary research tasks in this work package will be:
- Extract statistics from outcrops and analogue data.
- Define rules that are generally applicable, while still covering key elements.
- Formulate geological rules compatible with a mathematical formulation.
Obtaining geologically realistic output requires carefully designed rules for how events relate to each other. A rule may be absolute (“every object must have a non-negative thickness”) or approximate, expressing a frequently occurring pattern (“sediment tends to be deposited preferentially in local topographic lows”). We need a set of rules that allows smart choices of shape, size, orientation and other properties of the next event given all available information, including previously generated events as well as observations.
Output from this work package will be useful for all the other work packages. The rules will be used in the Bayesian model specification (Q2), they guide which parameters should be extracted from analogue data (Q3), and can help the design of the prior model specification (Q4). Since the development in Q4 requires generating conditional facies realisations reasonably fast, we will have to find the appropriate balance between rules that are geologically desirable versus rules that are computationally manageable.
Q2 - How can we specify geological rules in a Bayesian statistical framework?
The main goal of this work package is to formalise the use of geological rules into the Bayesian framework. Success for this work package is to deliver a coherent Bayesian framework for honouring both geological rules and reservoir data.
Primary tasks in this work package will be
- Integration of geological rules as a modelling constraint in the Bayesian framework.
- Explicit mathematical formulation of geological «rule of thumbs».
- Honouring of geological rules when conditioning to reservoir data.
The research tasks will be to make a fully integrated model specification and theoretical validation of an associated algorithm for Bayesian posterior assessment. This work also involves evaluating the practical application of the algorithm when applied in a workflow conditioning to well data and production data. We will start by looking at how a statistically consistent prior model can be built from geological rules. A range of statistical models will be considered; for instance, a stacking pattern can be seen as both a point process and as a hierarchical model. The rules should be geologically sound, not too complex mathematically and parameters should be either meaningful for geologists, possible to estimate from data, or both. Moreover, part of the tasks will be to formulate the model so that it fits in ensemble-based workflows. That may require extensions of the ensemble smoother, such as an ability to handle models of different rank.
For conditioning, we need to specify a data likelihood. Doing this for facies models is a well understood problem. In this project, we will also formulate a likelihood for outcrop observations, which will allow full integration of these in the modelling. The choice of likelihood functions can have great impact on convergence of posterior sampling algorithms, so even though this is the key focus of Q4, it is important to be aware of these issues when formulating the model. The results of this work package will be used to assist the implementation in Q4. There will be an interplay between the set of rules that are wanted (Q1) and statistically consistent (Q2).
Q3 - Which geostatistical input parameters can be estimated from geological analogues?
The goal of this work package is to find what parameters are well suited for numerical quantification and be able to estimate distributions for various analogues. The success criteria for this work package is the development of an algorithm to estimating input values from the database that can be used in the facies model building process.
Primary tasks in this work package will be
- Identify and extract relevant parameters for the reservoir facies model, such as contact relationships and object geometries.
- Translate from visual interpretation of outcrops to numerical data.
- Develop an algorithm to estimate the distributions of relevant parameters.
The SAFARI database is a natural starting point for extracting parametric data, being a world-leading outcrop analogue collection used and sponsored by numerous industry partners operating in Norway. The deposition of facies depends on a number of parameters such as depositional direction, depositional environment, sand-supply, relative sea-level changes and available accommodation space. This work package will ensure that the set of geological rules are compatible with parameters that can be extracted from analogue databases. Important parameters to consider are facies geometries and sand fraction, which in turn is indirectly controlled by wave-climate, shelf gradient and shoreline trajectory for a shallow marine system.
The result of this work package will provide feedback to the rule definitions (Q1), the likelihood model (Q2) and parameterisation choices in the model design (Q4).
Q4 - How to achieve convergence of a facies model conditioned to geological rules and data?
This task is the dominant work package of the project, and it is also critical for the overall project success. This work package is where the outcome of the other research tasks will be synthesized and taken into account as the project progresses. The success criteria of this work package is to have an executable program that produces realistic 3D facies realisations conditioned to reservoir data within reasonable simulation time. The major challenges are (a) that even simple rules will give very slow simulations, (b) that rules for depositional environments are not general enough, and there will be many exceptions to the rule to produce realistic models, and (c) that well conditioning in the presence of rigid rules will have problems converging. We have seen these types of challenges before in previous facies model development projects. We will therefore rely on the competence and experience in finding practical solutions to mitigate these risks, such as finding solutions to optimise well conditioning algorithms, making more informed choices for the prior model or simplifying the requirements imposed by the rules.
The main tasks in this work package will be:
- Create a prior model consistent with the geological conceptual model.
- Implement well conditioning algorithms for vertical and horizontal wells.
- Balance honouring geological rules and conditioning to reservoir data in a practical implementation.
- Ensure compatibility with industry workflows through parameterisation choices and supporting industry formats.
The novelty of the GEOPARD project lies in expanding the core of the rigorous Bayesian statistical framework to include geological conceptual rules. Our ambition is to design a rule-based algorithm with a solid theoretical foundation, which also works in practice. By working in practice we mean that it can be run in multi-realisation workflows applied by the industry. The new facies modelling algorithm allow for efficient screening, and can reveal inconsistencies between input data and the specified model. Such feedback can improve understanding of the underlying geological systems, which in turn impacts reservoir decisions. If successful in keeping geological realism when conditioning the model to production data in assisted history matching workflows, it will be a unique solution to a long-standing problem for the ensemble-based data assimilation approach.
Images
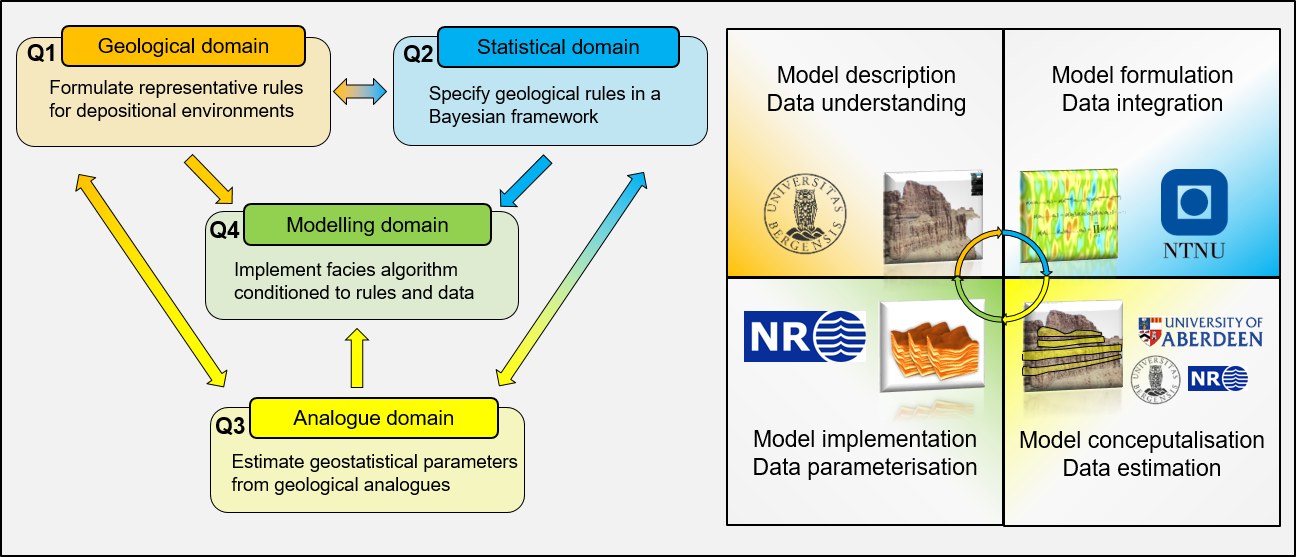
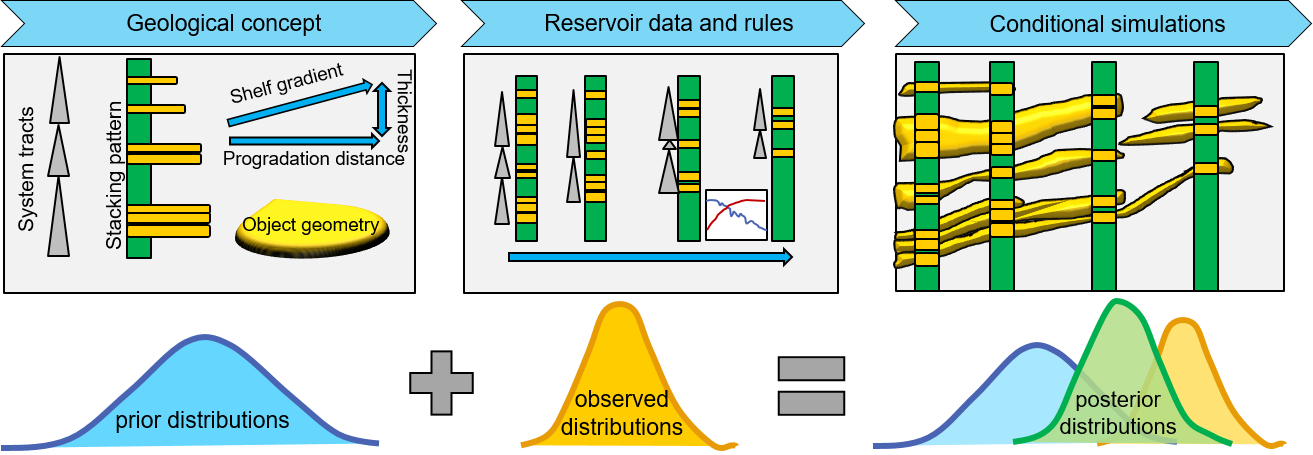
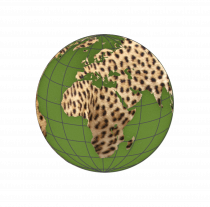
Project period
Financing
PETROMAKS2 / NFR
Equinor Energy AS
Winthershall Dea Norge AS
AkerBP ASA
Partners
University of Bergen
NTNU Trondheim
University of Aberdeen
Equinor Energy AS
Winthershall Dea Norge AS
AkerBP ASA